CropMapper-Ferghana
Using satellite remote sensing and cloud-based unsupervised machine learning, hydrosolutions GmbH has implemented a fully automatic mapping procedure for the global drylands that allows to identify irrigated land, crops and water consumption.
resolution crop maps
resolution evapotranspiration maps
classification accuracy
years of data and many years to come
Using satellite remote sensing and cloud-based unsupervised machine learning, hydrosolutions GmbH has implemented a fully automatic mapping procedure for the global drylands that allows to identify irrigated land, crops and water consumption with the CropMapper web app at the tip of a finger at any place of your choice. Different versions of the CropMapper application have been implemented and validated in Uzbekistan, Iran, Ethiopia or Lesotho. The app brings together state-of-the art remote sensing techniques for crop type mapping and for evapotranspiration mapping. In combination with global precipitation datasets, the CropMapper derives the relevant indicators for assessing irrigation water requirements of each crop. The app can also be used for collecting agricultural statistics (e.g., the development of crop type areas over time), and of course for generating, visualizing, and downloading high-resolution (10 m) agricultural maps.
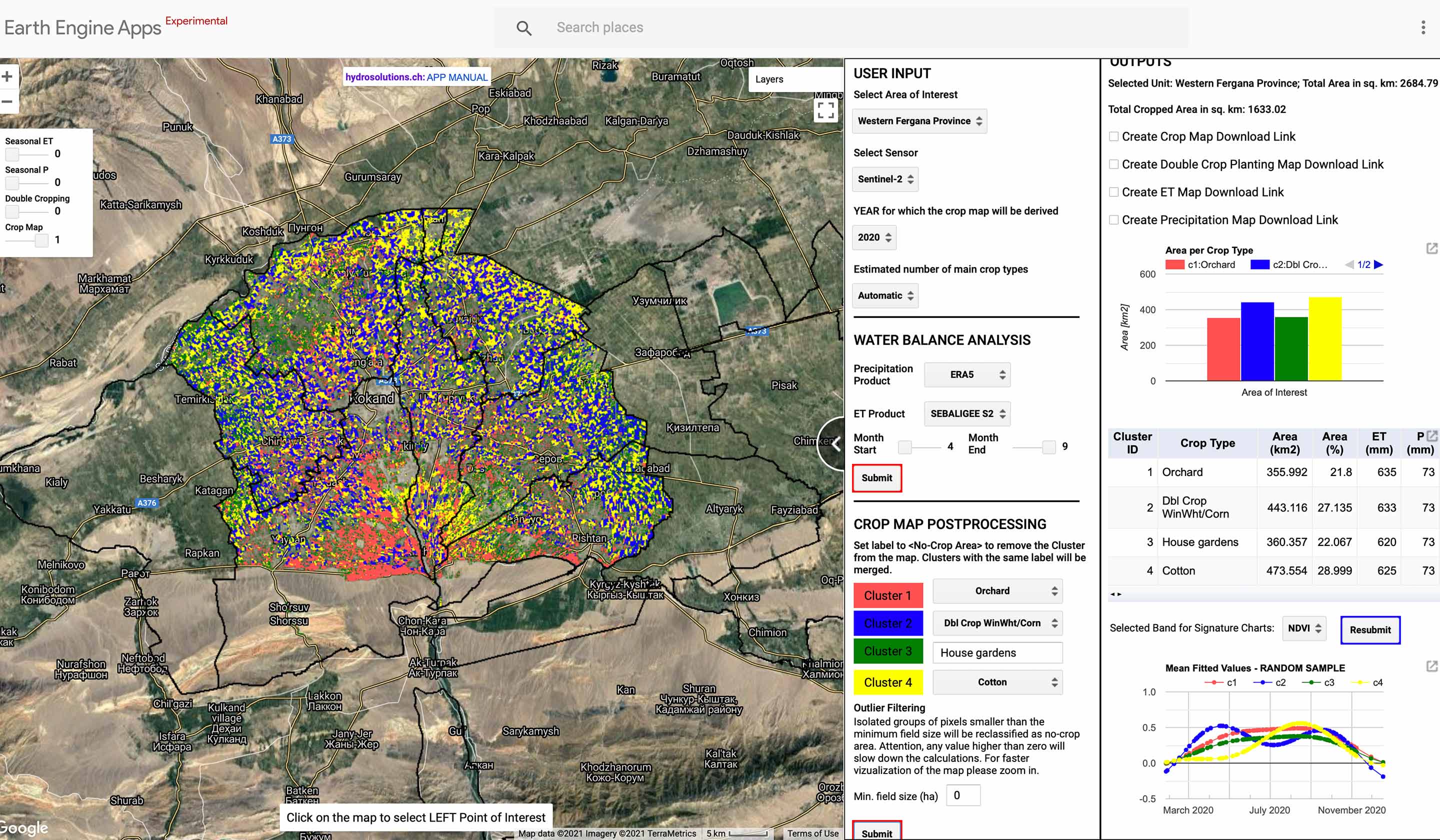
The big advantage of the CropMapper app is that all variables are identified based on remote sensing and reanalysis data. Data points collected in the field or crop statistics are not required as an input. This guarantees a fully automatic workflow and results available immediately after then end of each crop growing season. The crop type mapping algorithm is based on an unsupervised classification of land use. The machine learning algorithm trains itself to first identify the number of main crops in a region for a given year and then assigns a label to every pixel. Since the algorithm is entirely unsupervised, the crop names are not known a priori. The app therefore requires the user to modify these labels by assigning a crop name to every initial label.
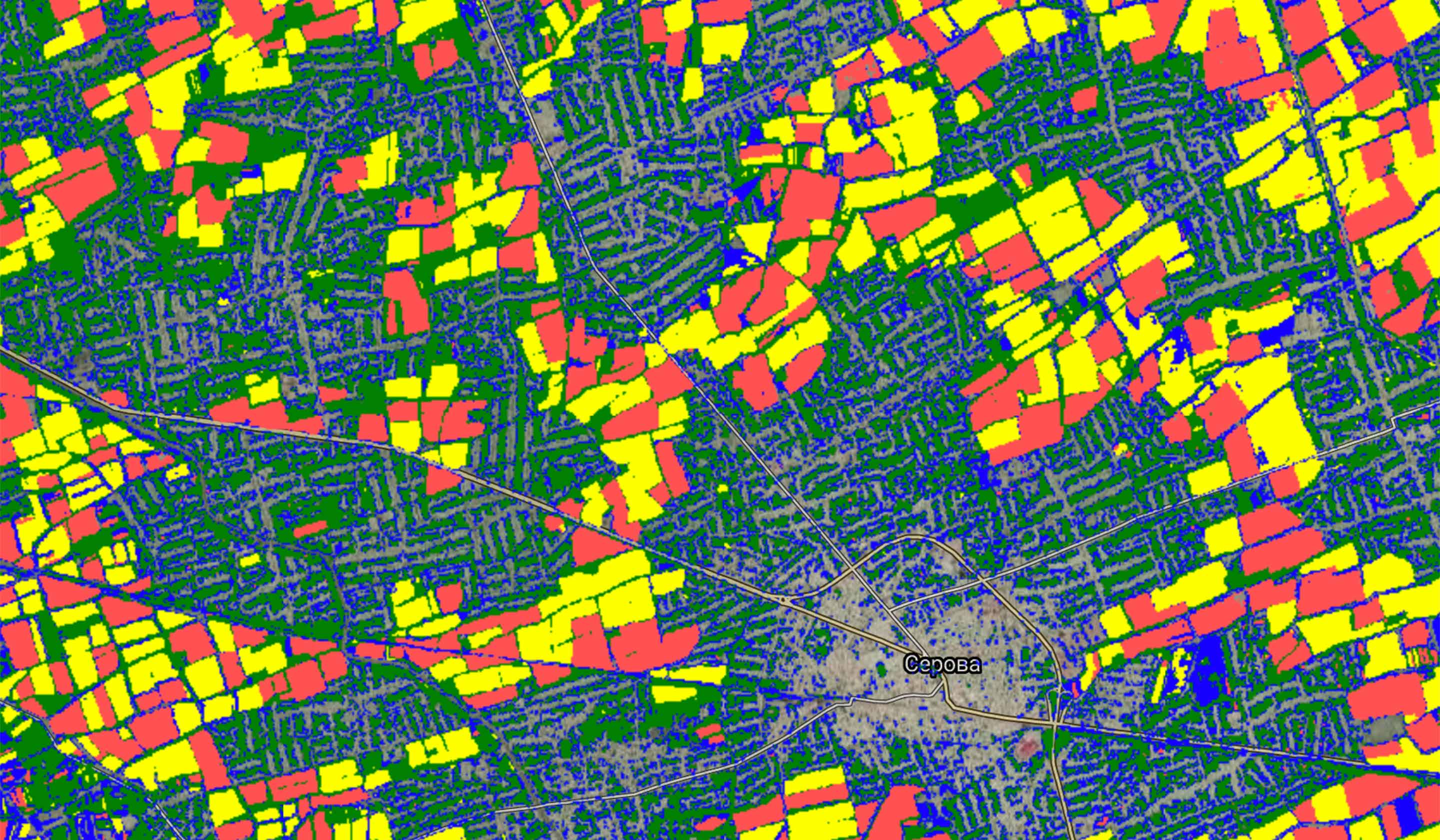
Central Asia is a particularly suitable place for remotely sensed monitoring of agriculture because of the usually cloud-free conditions during the crop growing period. The app therefore relies entirely on optical remote sensing imagery such as provided by Sentinel-2 or the Landsat satellites. For regions with frequent cloudy or hazy conditions we recommend a different approach that combines optical and radar remote sensing imagery.